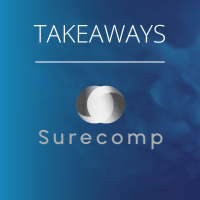
Since ChatGPT, which is a type of Generative AI, came out in 2022, many businesses have been exploring how to use this new technology in their operations—including treasury tasks. Generative AI is pre-trained on vast amounts of data and can create new content such as text, images or even code. It generates outputs based on patterns it has learned but doesn’t necessarily understand the specific context or details of treasury management.
While there’s a lot of excitement around generative AI, I haven’t seen many practical applications for it in treasury yet.
On the other hand, traditional AI has been around for much longer and has already found its place in treasury operations. This type of AI is designed for specific tasks and is trained on specialized datasets. It excels at pattern recognition, data analysis and automating routine processes within a defined scope.
There have been notable successes with this type of AI in corporate treasury:
Cash flow forecasting
AI has proven to be very useful for some companies in predicting cash flow. For example, I have come across success stories from several companies. Pearson, an educational company, has used AI models to dig deep into their cash flow forecasts. They compare their predicted numbers with the actual results to find out why there were differences. By feeding this information back into the AI model, it learns and gets better over time. This helps treasury teams plan cash more accurately without having to adjust things manually.
Similarly, Ingka Group (IKEA) has successfully used custom-built AI for better cash flow forecasting. This is encouraging because it shows that companies can develop their own AI solutions tailored to their specific needs. Traditional AI models, trained on the company’s data, can recognize patterns and make predictions that are highly relevant to their specific operations.
Fraud Detection
AI has also been helpful for some companies in detecting fraud. It can analyze large amounts of transaction data to find unusual patterns that might indicate fraudulent activity. Companies like KPMG have used AI to flag suspicious payment patterns that would be hard for people to spot on their own. This improves security without adding extra work for treasury teams.
Automating receivables management
AI can also help in automating processes like receivables management, which helps with working capital.
In one case study, a company dealing with thousands of monthly checks was struggling with manual processing delays and low team morale. They implemented an AI solution that automated the matching of payments to invoices, even when customers paid in complex ways. This led to faster payment processing—most payments were handled within two days—improved cash flow, and allowed the team to focus on more valuable tasks. As a result, the company enhanced its working capital by getting cash into the business more quickly.
Written by Jan-Willem Attevelt Co-Founder of Automation Boutique (Source)